"Leonardo has shown excellent scientific skills and high productivity. During his PhD, he had the opportunity to spend a few months at two of the greatest research centers of the Country: the NASA Jet Propulsion Laboratory (JPL) in Pasadena, California and the National Weather Center (NWC) in Norman, Oklahoma."
Viviana Maggioni, assistant professor, Sid and Reva Dewberry Department of Civil, Environmental, and Infrastructure Engineering
Story Update
Since graduating in 2018, Leo has accepted a job with Radar Expert at the Swedish Meteorological and Hydrological Institute (SMHI) in Norrköping, Sweden.
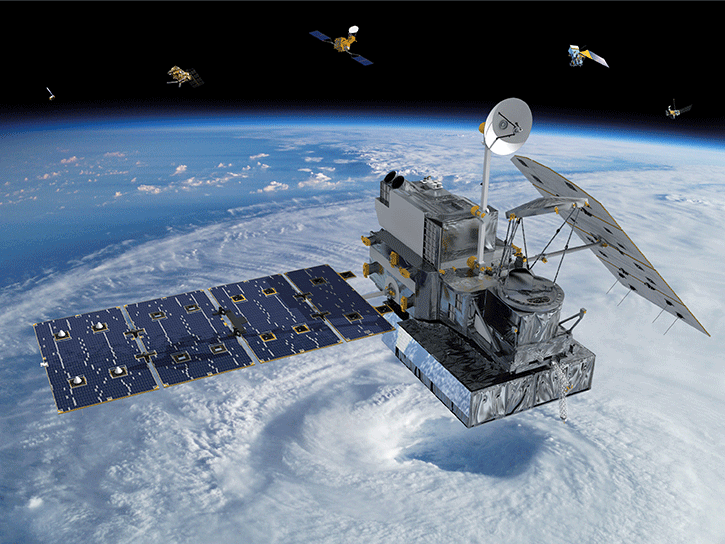
Visualization of the GPM Core Observatory and partner satellites. Porcacchia's classification scheme can be applied to the dataset collected by space-borne radar and can detect collision-coalescence processes at a global scale. (Photo credit: NASA.gov)
Leonardo Porcacchia remembers watching the news when he was growing up in Italy and seeing reports about weather hazards. As a young boy he saw big cities, small communities, and mountainous regions devastated by natural disasters caused by floods and landslides. He says the scenes and stories from his youth drove him to become a scientist of the atmosphere with focus on precipitation.
Extreme weather events represent a serious problem not only in Italy, but everywhere in the world, particularly in remote regions that are not well equipped with instruments to predict these events and prepare for them.
Porcacchia’s research focuses on a peculiar precipitation process in the atmosphere, a process responsible for high intensity rainfall rates at ground level.
“The process, called collision-coalescence, is like the snowball effect, except for rain drops. It works like this, some drops in the cloud reach the point where they start to fall towards the surface,”he says. “In their descent, if the right conditions are met, they collide with smaller drops, collect them, and become larger. It is like a snow ball rolling down a hill and collecting fresh snow and becoming bigger and bigger.”
Collision-coalescence processes are very effective at increasing the amount of water that falls to the ground and may lead to flash floods, but they are often difficult to detect. That’s why Porcacchia has been developing a classification scheme for identifying collision-coalescence scenarios that use observations from advanced radar to see inside clouds.
Recently a new satellite mission dedicated to precipitation has been launched. The Global Precipitation Measurement (GPM) is a NASA-JAXA (Japanese Aerospace Exploration Agency) satellite mission to provide next-generation observations of rain and snow worldwide every three hours.
The GPM Core Observatory satellite carries advanced instruments that set a new standard for precipitation measurements from space, including a Dual-frequency Polarimetric Radar (DPR). The data they provide is used to unify precipitation measurements made by an international network of partner satellites to quantify when, where, and how much it rains or snows around the world.
“The GPM mission contributes to advancing our understanding of Earth's water and energy cycles, improves the forecasting of extreme events and extends current capabilities of using satellite precipitation information to directly benefit society,” says Viviana Maggioni, assistant professor in the Sid and Reva Dewberry Department of Civil Environmental, and Infrastructure Engineering, and Porcacchia’s advisor. “ Leonardo’s research aims at improving the DPR algorithm for precipitation estimation.”
“My classification scheme can be applied to the dataset collected by this space-borne radar and therefore I am able to detect collision-coalescence processes at a global scale,” Porcacchia says.
“One of the significant limitations of remotely sensed data is the potential error associated with the data, especially in the case of precipitation systems triggered by orography, which yield significant non-meteorological backscatter of the radar signal,” says Maggioni. “Leonardo’s work proposes a new algorithm to improve the classification of precipitation regimes observed by radars and, consequently, improve the estimation of rainfall rates to reduce those errors and uncertainties.”
Porcacchia thinks his classification scheme and this space-borne radar dataset together will improve real-time prediction of rainfall rates in case of extreme events and help remote regions all over the world that cannot count on local ground instruments. Particularly, it will help communities like those in Italy that first inspired his work.